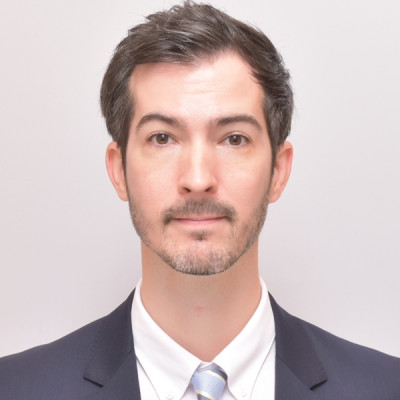
Michael McGuire
Doshisha University, Department of English
About
No profileSessions
Presentation The Current State of Automatic Speech Recognition for Non-Native English more
Automatic Speech Recognition (ASR), or the automated conversion of spoken language into text, is an essential component of computer assisted language learning (CALL) and computer assisted language testing (CALT). However, ASR is a rapidly developing technology and has reached its highest levels of accuracy in the past few years thanks to advances in neural networks and transformer systems. This study looks at five state-of-the-art ASR systems (AssemblyAI's Universal-2, Deepgram's Nova-2, RevAI's V2, Speechmatics' Ursa-2, and OpenAI's Whisper-large-v3) and measures their accuracy on non-native accented English speech from six different L1 backgrounds, in the form of both 2400 read sentences and 22 spontaneous narrative recordings. Results found that all systems achieved mean Match Error Rate (MER) of less than 0.09, or above 91% accuracy on read speech. Two systems performed especially well, with no significant difference found between them: Whisper had the lowest mean MER of 0.054 followed by AssemblyAI with 0.056. For spontaneous speech, RevAI had the lowest mean MER of 0.074. All five systems performed better than other ASR systems reported on in the last several years, suggesting that accurate transcription of non-native English speech is possible.
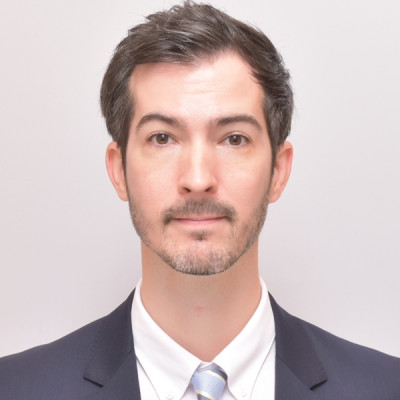