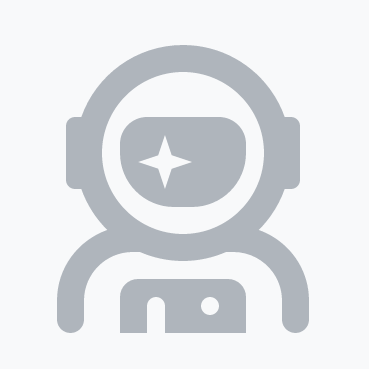
Joe Geluso
About
No profileSessions
Presentation Exploring the Propositional Content of a Corpus: A Comparative Case Study of Methods in Keyword Analysis and Topic Modeling more
Sun, Jul 20, 09:00-09:25 Asia/Tokyo
Keyword analysis is a popular method for text analysis as it identifies words that reflect the propositional content of a group of texts when compared to a reference corpus. Recently, topic modeling has been increasingly used for similar purposes as it identifies thematic groupings of words that co-occur within and across the texts, but unlike keyword analysis, words are identified independently from a reference corpus. In this presentation, findings from quantitative metrics and qualitative methods used to compare and contrast the output of two methods of keyword analysis (i.e., log-likelihood and t-test) and topic modeling are shared. It was found that keyword analysis utilizing log-likelihood provides an accurate overview of the propositional content of texts with a strong focus on content words. Wordlists generated via the t-test demonstrated substantial overlap with log-likelihood, but featured comparatively more function words reflecting academic writing conventions. Finally, words generated by topic modeling had more in common with the log-likelihood keywords than the t-test, and benefitted from the grouping of co-occurring words and independence from a reference corpus. These findings have implications for CALL, as corpus-based text analysis can support vocabulary development and academic writing by providing learners with targeted and discipline-appropriate linguistic insights.
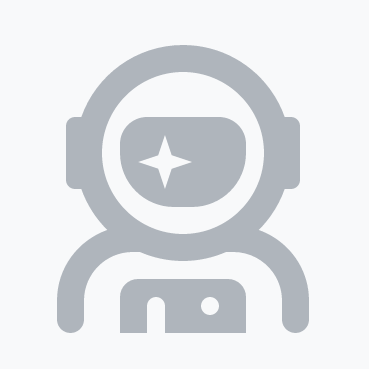